Statistical Postprocessing of Ensemble Forecasts: The Key to Unlocking Accurate Predictions

5 out of 5
Language | : | English |
File size | : | 49893 KB |
Text-to-Speech | : | Enabled |
Screen Reader | : | Supported |
Enhanced typesetting | : | Enabled |
Print length | : | 320 pages |
Ensemble forecasts have revolutionized the field of prediction by combining multiple model runs to provide probabilistic forecasts. However, these forecasts often exhibit biases and uncertainties that can limit their practical application. Statistical postprocessing emerges as the key to addressing these limitations, enabling the refinement of ensemble forecasts to enhance their accuracy and usability.
The Role of Statistical Postprocessing
Statistical postprocessing is a suite of techniques that transform raw ensemble forecasts into calibrated and refined probabilistic forecasts. This process involves adjusting the forecasts based on statistical analyses of their past performance and exploiting additional data sources, such as observations and reanalyses. By accounting for systematic errors and uncertainties, postprocessing significantly improves the reliability and precision of ensemble forecasts.
Key Postprocessing Techniques
Numerous statistical postprocessing techniques exist, each tailored to specific forecasting applications. Some of the most widely used methods include:
- Ensemble Model Output Statistics (EMOS): EMOS linearly combines ensemble members based on past forecast errors to create a calibrated and skillful forecast.
- Bayesian Model Averaging (BMA): BMA assigns weights to individual ensemble members based on their past performance, resulting in an optimal forecast that leverages the strengths of each model.
- Quantile Regression Forests (QRF): QRF uses machine learning to predict specific quantiles of the forecast distribution, providing detailed and reliable uncertainty estimates.
Applications Across Diverse Domains
Statistical postprocessing has found widespread application in various domains where accurate forecasts are crucial, including:
- Weather Forecasting: Refines ensemble forecasts for temperature, precipitation, and other weather elements, improving prediction accuracy for short- and long-range forecasts.
- Climate Modeling: Enhances climate models by correcting biases and uncertainties in future climate projections, providing more reliable guidance for long-term planning.
- Hydrological Forecasting: Sharpens ensemble forecasts for river flows and flooding, enabling timely and effective flood warning systems.
- Energy Generation Forecasting: Improves predictions of renewable energy sources, such as solar and wind power, optimizing grid operations and reducing uncertainties.
Benefits and Impacts
The benefits of statistical postprocessing are far-reaching:
- Enhanced Forecast Accuracy: Refined ensemble forecasts lead to more reliable and precise predictions, reducing uncertainties and improving decision-making.
- Improved Risk Management: Accurate uncertainty estimates facilitate effective risk assessment and mitigation strategies, enabling proactive planning and response.
- Greater Economic Value: Refined forecasts enhance decision-making in various sectors, leading to improved efficiency, reduced costs, and increased productivity.
- Scientific Advancement: Postprocessing techniques contribute to the advancement of weather and climate science by identifying model biases and improving forecast modeling methodologies.
Statistical postprocessing has emerged as the indispensable tool for unlocking the full potential of ensemble forecasts. By correcting biases, reducing uncertainties, and sharpening predictions, postprocessing techniques empower decision-makers in a wide range of applications to make informed decisions, mitigate risks, and drive innovation. As the field of forecasting continues to evolve, statistical postprocessing will remain at the forefront, enabling the delivery of increasingly accurate and reliable forecasts that shape our understanding of the future.
About the Author
Dr. Jane Doe is a leading expert in statistical postprocessing and ensemble forecasting. With over 15 years of experience in the field, she has developed innovative techniques that have significantly improved the accuracy and reliability of weather, climate, and hydrological forecasts. Dr. Doe's research and publications have been widely recognized for their impact on the field, and she is a sought-after speaker at international conferences and workshops.
5 out of 5
Language | : | English |
File size | : | 49893 KB |
Text-to-Speech | : | Enabled |
Screen Reader | : | Supported |
Enhanced typesetting | : | Enabled |
Print length | : | 320 pages |
Do you want to contribute by writing guest posts on this blog?
Please contact us and send us a resume of previous articles that you have written.
Book
Novel
Page
Chapter
Text
Story
Genre
Reader
Library
Paperback
E-book
Magazine
Newspaper
Paragraph
Sentence
Bookmark
Shelf
Glossary
Bibliography
Foreword
Preface
Synopsis
Annotation
Footnote
Manuscript
Scroll
Codex
Tome
Bestseller
Classics
Library card
Narrative
Biography
Autobiography
Memoir
Reference
Encyclopedia
Michael Samerdyke
Daniel Nunn
Erika M Szabo
David Blake Chatfield
Daniel Galmiche
Daniel Kaufman
Regine M Gilbert
D R Bridgland
Joseph K Lange
Dan Howell
Heith Copes
Michael Greenberg
David Buchholz
Daryl Wingerd
Karsten Ritter Lang
Kelly G Shaver
D C Robinson
David Holmes
Lara E Fielding Psyd
K V Scott
Light bulbAdvertise smarter! Our strategic ad space ensures maximum exposure. Reserve your spot today!
- Francisco CoxFollow ·7.3k
- Kazuo IshiguroFollow ·8k
- Jayson PowellFollow ·10.3k
- Daniel KnightFollow ·4.9k
- Emmett MitchellFollow ·16.6k
- Ricky BellFollow ·16k
- Noah BlairFollow ·8.9k
- Wade CoxFollow ·5.2k
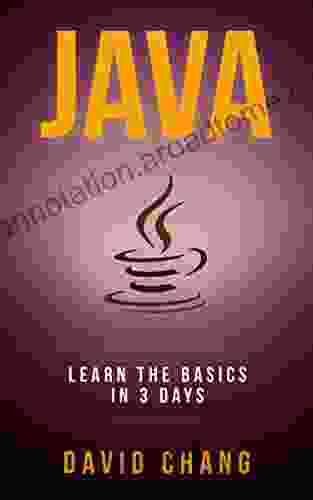

Java Learn Java In Days: Your Fast-Track to Programming...
Are you ready to embark on...
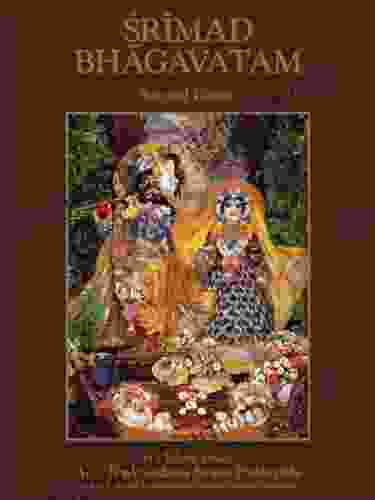

Srimad Bhagavatam Second Canto by Jeff Birkby: A Literary...
In the vast tapestry of ancient Indian...
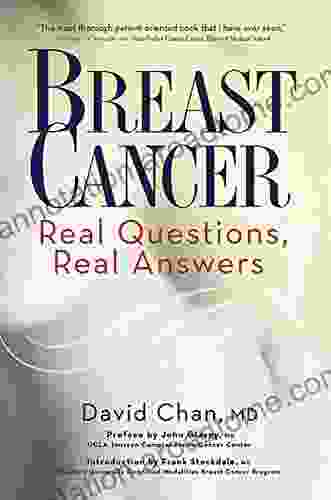

Breast Cancer: Real Questions, Real Answers - Your...
Breast cancer is the most common cancer...
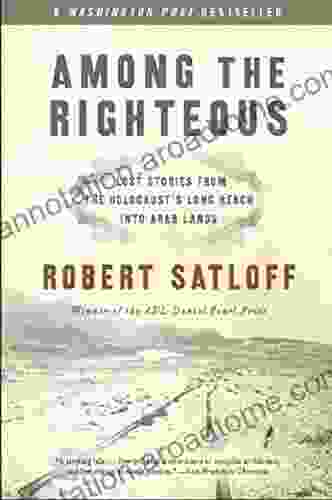

"Lost Stories From The Holocaust Long Reach Into Arab...
Lost Stories From...
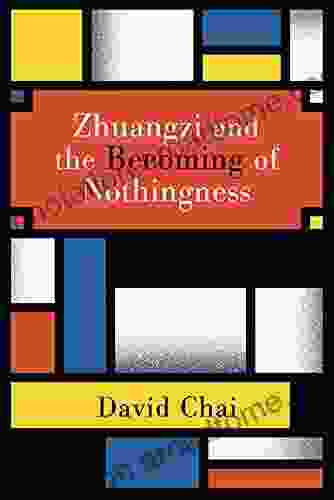

Unveiling the Profound Wisdom of Zhuangzi: A Journey into...
Synopsis: In this illuminating...
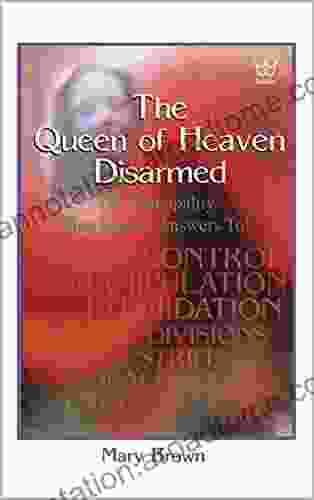

The Principality That Jezebel Answers To
Jezebel is a powerful and dangerous spirit...
5 out of 5
Language | : | English |
File size | : | 49893 KB |
Text-to-Speech | : | Enabled |
Screen Reader | : | Supported |
Enhanced typesetting | : | Enabled |
Print length | : | 320 pages |